Blog
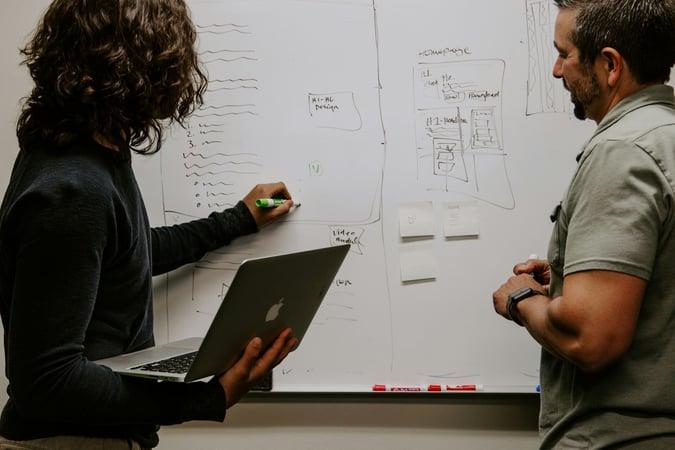
The vital importance of data governance in the age of AI
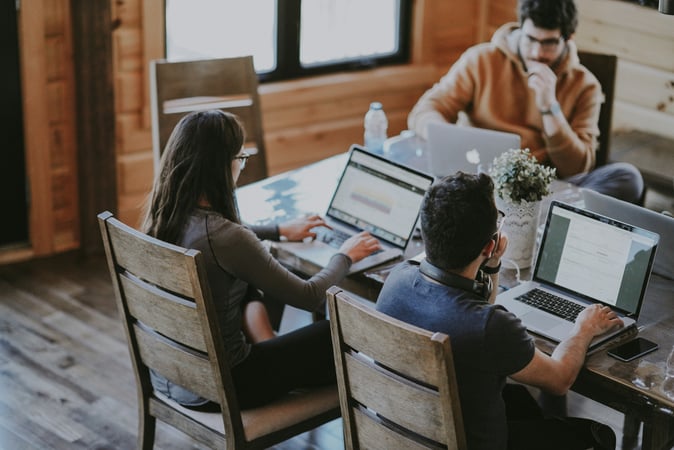
Bringing a human perspective to data integration, mapping and AI
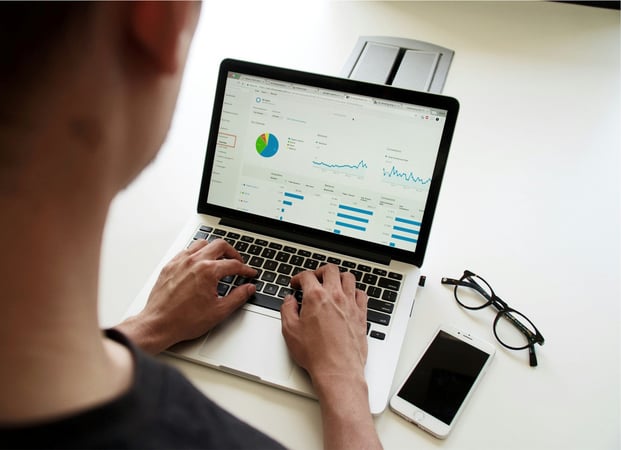
How AI is shaping the future of data integration
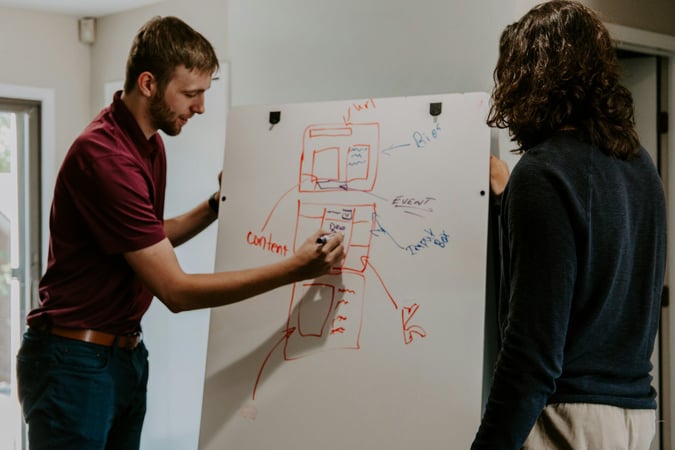
How to say ‘yes’ to all types of data and embark on a data-driven transformation journey
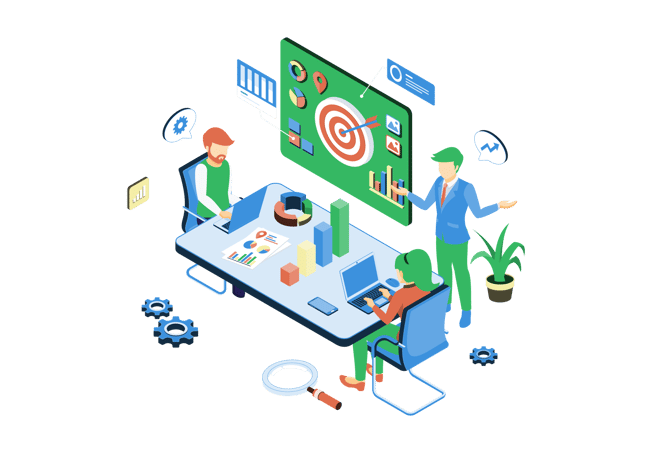
Data ingestion tools: 7 features you should look for
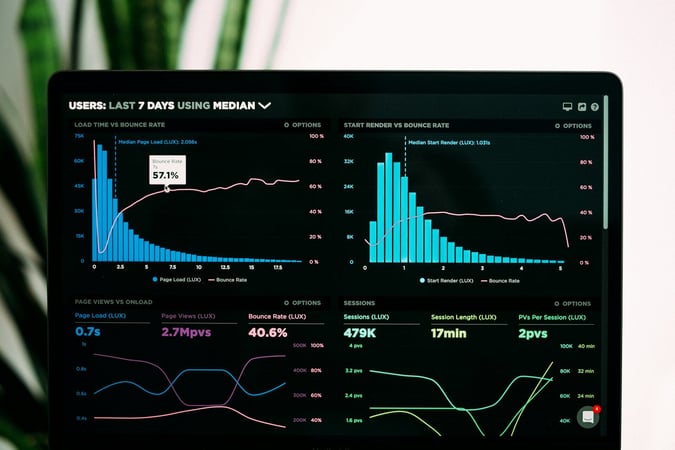
Data literacy, visualization and the challenges of data integration
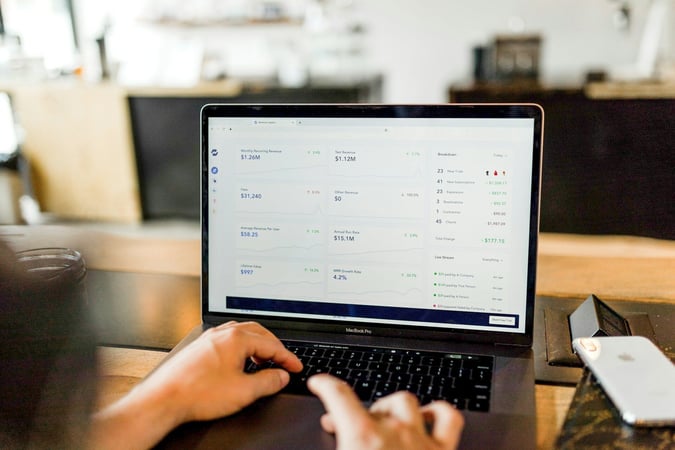
Why data quality is crucial for data integration projects
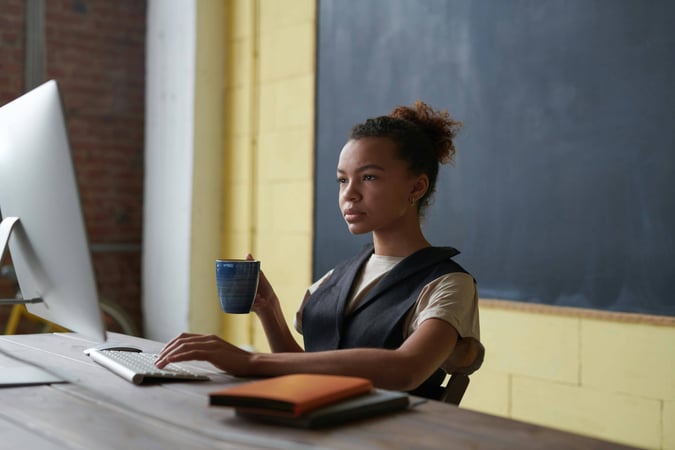
Choosing the right data integration software: 12 essential questions
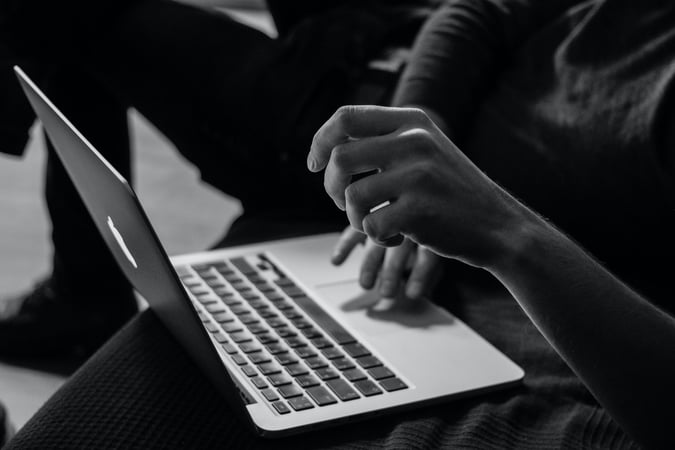