What is data ingestion?
Data ingestion is the process of moving or onboarding data from one or more data sources into an application data store. Every business in every industry undertakes some kind of data ingestion - whether a small scale instance of pulling data from one application into another, all the way to an enterprise-wide application that can take data in a continuous stream, from multiple systems; read it; transform it and write it into a target system so it’s ready for some other use.
What's the difference between data ingest and data migration?
A data migration is a wholesale move from one system to another with all the timing and coordination challenges that brings. Migration is often a ‘one-off’ affair, although it can take significant resources and time.
Data ingestion on the other hand usually involves repeatedly pulling in data from sources typically not associated with the target application, often dealing with multiple incompatible formats and transformations happening along the way.
Types of data ingestion
There’s two main methods of data ingest:
- Streamed ingestion is chosen for real-time, transactional, event-driven applications - for example a credit card swipe that might require execution of a fraud detection algorithm.
- Batched ingestion is used when data can or needs to be loaded in batches or groups of records. Batched ingestion is typically done at a much lower cadence but with much higher efficiency.
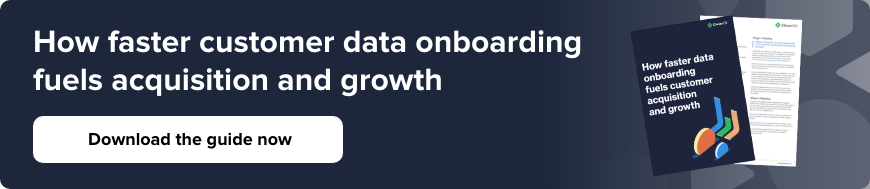
The data ingestion process
The process of data ingestion consists of several steps.
- Detect that new data is available to onboard: Whether files are sent via email, or dropped into an FTP site, your ingestion pipeline should detect these automatically and move them for processing.
- Inspect the layout and format of that data: There can be several layers to data validation in data ingestion, from checking that all necessary files are present, to quality checks on the data itself (is it the right format? Are there too many null values?)
- Read the data
- Map and transform data: The pipeline needs to know how to map the data to the target, but also what transformations are needed, such as combining or splitting up fields.
- Assess data quality: Validating data again helps keep quality high, and data can be validated against specific business rules to ensure that details are correct.
- Load data to target: Once files are validated, transformed and ready to go, the next stage is pushing them to their destination, whether that's storage such as a data warehouse, or on to further processing.
- Detect issues and log progress through each step: Finally, your pipeline needs to create a log detailing how the process went, and any errors that occurred.
Steps of a data ingestion process
To streamline processes, save time on manual work and reduce errors, as much of your data ingestion process as possible should be automated.
Data ingestion examples
Data ingestion can take a wide variety of forms. These are just a couple of real-world examples:
- Ingesting a constant stream of marketing data from various places in order to maximize campaign effectiveness
- Taking in product data from various suppliers to create a consolidated in-house product line
- Loading data continuously from disparate systems into a data warehouse
Data ingestion case studies
Data ingest challenges
Setting up a data ingestion pipeline is rarely as simple as you’d think. Often, you’re consuming data managed and understood by third parties and trying to bend it to your own needs. This can be especially challenging if the source data is inadequately documented and managed.
For example, your marketing team might need to load data from an operational system into a marketing application. Before you start, you’ll need to consider these questions:
- Is the data to be ingested of sufficient quality? How do I define and measure the quality metrics?
- After the data has been ingested, is it usable ‘as is’ in the target application?
- If you’re ingesting data from various sources, what formats are you dealing with? And can your ingest platform handle them all?
- Is the data stream reliable and stable?
- What performance or availability levels, or SLAs, do you need to consider for your data or target system?
- How will you access the source data and to what extent does IT need to be involved?
- Is your engineering team likely to be a bottleneck to the process?
- How often does the source data update and how often should you refresh?
- Prior to the ingestion process beginning, are you confident that your data is high-quality and that you have robust data validation in place?
- How will the process be automated?
Setting up a data ingestion pipeline
Automating data ingest
When you’re dealing with a constant flow of data, you don’t want to have to manually supervise it, or initiate a process every time you need your target system updated. You really want to plan for this from the very beginning otherwise you'll end up wasting lots of time on repetitive tasks.
Human error can lead to data integrations failing, so eliminating as much human interaction as possible can help keep your data ingest trouble-free. (This is even more important if the ingestion occurs frequently).
Both these points can be addressed by automating your ingest process.
You’ll also need to consider other potential complexities, such as:
- A need to guarantee data availability with fail-overs, data recovery plans, standby servers and operations continuity
- Setting automated data quality thresholds
- Providing an ingest alert mechanism with associated logs and reports
- Ensuring minimum data quality criteria are met at the batch, rather than record, level (data profiling)
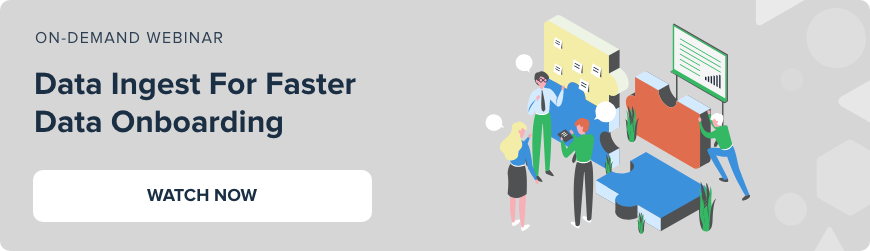
Data ingest can also be used as a part of a larger data pipeline. Other events or actions can be triggered by data arriving in a certain location. For example - a system that monitors a particular directory or folder, and when new data appears there, a process is triggered.
Data ingestion parameters
There are typically 4 primary considerations when setting up new data pipelines:
- Format – what format is your data in: structured, semi-structured, unstructured? Your solution design should account for all of your formats.
- Frequency – do you need to process in real-time or can you batch the loads?
- Velocity – at what speed does the data flow into your system and what is your timeframe to process it?
- Size – what is the volume of data that needs to be loaded?
It’s also very important to consider the future of the ingestion pipeline. For example, growing data volumes or increasing demands of the end users, who typically want data faster.
Governance and safeguards
Another important aspect of the planning phase of your data ingest is to decide how to expose the data to users. Typical questions asked in this phase of pipeline design can include:
- Will this be used internally?
- Will this be used externally?
- Who will have access to the data and what kind of access will they have?
- Do you have sensitive data that will need to be protected and regulated?
These considerations are often not planned properly and result in delays, cost overruns and increased end user frustration.
Real-time or batch ingest?
It’s important to understand how often your data needs to be ingested, as this will have a major impact on the performance, budget and complexity of the project.
There is a spectrum of approaches between real-time and batched ingest. For example, it might be possible to micro-batch your pipeline to get near-real-time updates, or even implement various different approaches for different source systems.
Understanding the requirements of the whole pipeline in detail will help you make the right decision on ingestion design.
The decision process often starts with users and the systems that produce that data. Typical questions that are asked at this stage include:
- How frequently does the source publish new data?
- Is the source batched, streamed or event-driven?
- Does the whole pipeline need to be real-time or is batching sufficient to meet the SLAs and keep end users happy?
Data ingestion with CloverDX
Your data ingestion process should be efficient and intuitive, and CloverDX’s automation capabilities can play a crucial role in this, giving you:
- Automated, transparent data pipelines for quicker customer data ingestion
- A simplified onboarding process, reducing reliance on development teams
- The capability to handle diverse data formats and sources, easing customer data preparation
- Efficient engineering, preventing bottlenecks in onboarding - as well as the ability to move work away from the engineering team to less technical colleagues
- Scalability without additional headcount, and the ability to handle large data volumes
- Automated error handling and validation for accurate data processing
Many businesses have improved their ingest processes with CloverDX, including helping clients free up a third of engineer time with data automation and triple their customer base without adding resource.
What the process looks like
Data ingestion encompasses various challenges and goals that are unique to your business. The first thing we do is learn about your specific challenges and what you want to achieve from the process. Some questions we will consider in this discovery stage will include:
- What specific goals do you aim to achieve through data ingestion?
- What are your data sources?
- What is the expected volume and variety of data?
- How frequently will data ingestion occur?
- How do you plan to integrate the ingested data with existing systems?
- What data quality checks are you expecting?
- Are there specific security or compliance requirements for the data?
- How do you foresee the data ingestion process scaling over time?
- How will you measure the success of the data ingestion process?
By seeking out the challenges and pain points unique to you we can help you conceptualize and build out your ideal automated data pipeline, empowering you to onboard data faster and deliver value sooner.
Read more about how the CloverDX Data Integration Platform can help with data ingest challenges.
Data Ingest with CloverDX
We can help
Our demos are the best way to see how CloverDX works up close.
Your time is valuable, and we are serious about not wasting a moment of it. Here are three promises we make to everyone who signs up:
- Tailored to you. Every business is unique. Our experts will base the demo on your unique business use case, so you can visualize the direct impact our platform can have.
- More conversation than demonstration. Have a question? We can help. Volume of data, quality of data and scalability are just a few of the challenges that can arise during the data ingestion process. Whatever concerns or reservations you have, let us know.
- Zero obligation. We’ve all been there. You spend some time hearing about a product or service… and then comes the hard sell. Our team doesn’t ‘do’ pushy. We prefer honest, open communication that leaves you feeling informed and confident.
Get in touch for a personalized demo.
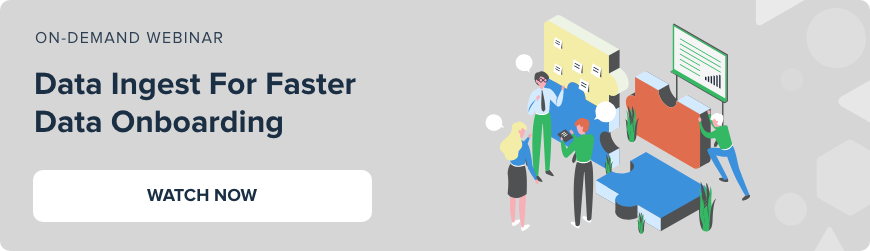